If you work in education, you’ve probably already mingled with terms like constructivism or names like Piaget. In employee training, instructional designers will make reference to Bloom’s taxonomy or Mayer’s work on multimedia learning. Learning theory can get decidedly academic, but that’s only because learning is a complex thing. Theories provide explanations for how and why we do things, and in order to design interfaces for learning that are genuinely effective, we need a basic understanding of how people go about learning new things.
Interestingly, different theories of how we learn can dramatically affect how a learning technology is designed. For example, will an eLearning program be designed with a single-user, linear architecture, or will it be open-ended and collaborative? That depends on your theory of learning.
So sit back and let’s dig into the essentials and academic name-dropping that will guarantee you success at any geek party of educational psychologists.
By the way, I don’t pretend this chapter is anything close to comprehensive. You couldn’t expect 150 years of science and philosophy to neatly condense into a few pages. Instead, I’ve selected the main theories at the core of 20th and 21st century learning with a focus on those that seem to crop up consistently in both corporate and school-based eLearning strategies.
Are You More of a Vessel or a Builder?
First, an esoteric interview question: Would you consider yourself an empty vessel or a construction worker? One basic distinction between how teachers and researchers think about learning has to do with whether they see learners as empty vessels into which knowledge is poured or as active builders of their own knowledge. Therefore, instruction becomes about how to best transfer knowledge to a learner’s brain or how to best facilitate opportunities for learners to construct their own knowledge.
In the first instance, the teacher is often described as a “sage on the stage,” and in the second, a “guide on the side,” or facilitator. Page-turner and multiple-choice courseware generally comes from the knowledge-transfer department. An open space with various tools for learners to seek out information, develop ideas, and share them with others comes out of the construction department.
Unpacking this a step further, we find that how you view learners depends on how you view knowledge. Is knowledge a collection of objective facts about the world that can be transferred? If so, you take an objectivist view. If you see human knowledge as something dynamic that is continually being adapted and constructed by people, individually and socially, then you take a constructivist view.
Views of Knowledge
Objectivist—Knowledge about the world is objective and gets transferred to a learner’s brain. The teacher is a “sage on the stage” who imparts knowledge.
Constructivist—Knowledge about the world is constructed by the learner. The teacher is a “guide on the side” who facilitates learning.
As you will see, the theories summarized in this chapter are like siblings—complete with shared history and the proverbial rivalry—and there are many ways in which they share and overlap. It is not my intention to suggest that one theory is better or more accurate than any another; nor are there clean and clear boundaries between them.
Surely, different perspectives on learning are valuable for different reasons and within different contexts; and no single theory is ideal for everything all of the time. I value the notion that by using different perspectives in complementary ways, we can get the best of all worlds.
Moreover, theories themselves are steps in a journey of discovery, not an end or a complete answer in themselves. With each step we broaden our understanding of what learning is, but we will always have more to discover.
Behaviorism: Learning as the Science of Behavior Change
The first major theory of learning, behaviorism, was born in the second half of the 19th century from animal behavioral studies. Behaviorism embraced what was the reasonably new concept of science. Behaviorists were adamant that the scientific method could and should be applied to the study and practice of learning and teaching.
In order to be scientifically precise about something as psychological and complex as learning, behaviorists claimed that only observable overt action—aka behavior—was worth studying because it is the only thing we can see and, therefore, measure empirically.
Furthermore, they believed that the inner workings of the mind, which occur in the “black box” of mental formations, were too airy fairy for real scientists to consider. They didn’t think our thoughts had much to do with causing our behavior and instead claimed all our behavior was triggered by external stimula.
In bringing a scientific approach to the study of learning for the first time, behaviorists sought to measure, predict, and manipulate patterns of behavior, using the now familiar notion of “stimulus – response” for research and training.
Behavioral Conditioning
The first big daddy of behaviorism is the celebrated Ivan Pavlov. Perhaps even more famous than Pavlov himself are his dogs. Knowing that dogs salivate in the presence of food, Pavlov conducted a legendary experiment in which he repeatedly rang a bell just before each mealtime. After a while, the dogs began to salivate in response to the bell itself, even when food never came. The dogs were conditioned to salivate in response to the bell.
In this famous experiment, Pavlov demonstrated the discovery that animals—including humans—could be conditioned to behave in certain ways on cue—by training one stimulus to trigger another (Figure 2.1). This is now known as classical conditioning.

At the turn of the last century, Edward Thorndike developed the notion of operant conditioning. Rather than working with the involuntary behaviors of classical conditioning—like salivating—Thorndike’s operant conditioning dealt with behaviors over which we have control. He also developed the conclusion, known as the “Thorndike Law of Effect,” which basically states that behaviors associated with pleasure and comfort are more likely to be repeated—“Mmmm, I’ll eat there again”—whereas those associated with displeasure are less likely to be repeated—“Brrr… No more sledding in my underwear.”
Thorndike’s studies formed the groundwork for the second big daddy of behaviorism, Burrhus Skinner. Skinner’s methods for operant conditioning relied on reinforcement and punishment, with an emphasis on positive reinforcement. Of course, Skinner didn’t invent the ideas of punishment and reward, but he conceptualized them scientifically and conducted experiments to determine how to use them most effectively, based on various schedules of frequency.
Reinforcement and Punishment
We’re all familiar with the notions of positive and negative reinforcement, but the latter is usually misunderstood. Positive and negative don’t mean pleasant or unpleasant, but whether something is being added or removed (+ or -).
Positive reinforcement is the easy one to remember as it refers to providing something nice as a reward for a desired behavior—like a dog getting a treat for rolling over. Negative reinforcement is still a reward because it involves removing something bad—like removing a dog’s muzzle when it stops growling.
Positive punishment is about adding something unpleasant—like clapping near the dogs ears when he barks—and negative punishment involves removing something pleasant—like not letting the dog sleep inside after he pees on the carpet. Both positive and negative reinforcement increase the likelihood of a behavior while punishments decrease it.
I use animal examples here—mainly because they’re easy to understand and dogs are cute—but indeed, Skinner’s experiments were largely on non-human subjects—he especially liked rats and pigeons. Many critics of behaviorism refer to the problematic nature of research on lab pigeons being applied to children in schools, and regard it as unsuited to supporting the breadth and complexity of human learning. Nevertheless, it remains one of the most easily recognized strategies at work in education today—even on the Web (Figure 2.2).

As politically incorrect as punishment has become for learning since the days of rulers on knuckles to encourage correct spelling behavior, behaviorist learning strategies continue to permeate our experience online and off. Fortunately, the methods are now less sadistic on the whole.
For example, in schools, we still get sent to detention (positive punishment) or excluded from playground games (negative punishment) for misbehaving. But reinforcement and punishment need not be as dramatic as they sound. More commonly, they are subtler cues and can be as simple as a teacher’s smile or frown that motivates a child.
Online, the consequences can be as subtle as color choice and wording. When Flickr praises you for uploading your photos “Good job!” or the Blackboard Learning Management System displays a confirmation in bright green that says “Success!” as if it’s celebrating with you for posting to a forum, you experience a small example of positive reinforcement.
Games and gamification often rely heavily on rewards. The so-called Foursquare technique consists of a series of positive reinforcements in the form of badges, points, levels, and leaderboards. Many of the most widely used eLearning programs and educational apps employ rewards like these to motivate learners (Figure 2.3).
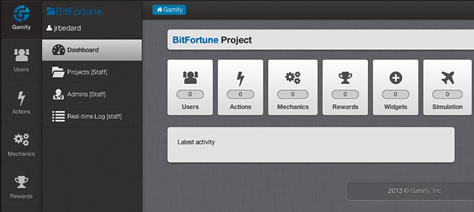
The critics of ill-founded attempts at gamification caution that these motivators are limited because they are entirely extrinsic. That is to say, a learner’s motivation to achieve a goal is based on external motivators (buttons and badges) and not on what they’re actually learning. But we’ll look at motivation in depth in the chapter on emotion.
Benjamin Bloom and His Taxonomy
Bloom and his taxonomy make regular appearances in seminars on instructional design. Bloom’s Taxonomy refers to a classification of learning objectives edited by Benjamin Bloom in 1956. Bloom was an American educational psychologist interested in applying scientific structure—akin to the classification of animals and plants—to learning goals in order to support educational design and assessment.
While Bloom envisioned a series of three taxonomies, it’s his cognitive taxonomy that was completed and which has become so widely applied. (The others were affective and motor-sensory.) It attempts to classify all possible cognitive learning objectives into five categories in order of lesser to greater cognitive complexity. These are: Knowledge, comprehension, application, analysis, synthesis, and evaluation.
Bloom’s five categories were later revised in 2001, leading to the following revamp.
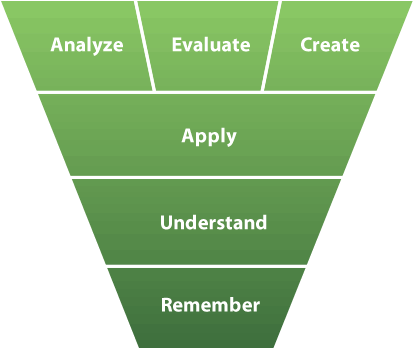
A revision of Bloom’s Taxonomy of cognitive learning objectives by Anderson, Krathwohl, and colleagues, 2001. It depicts remembering as a prerequisite for understanding and understanding as required for application.
Critics of Bloom’s taxonomy point out that many complex tasks involve many different processes going on in parallel. Tasks should not be created for only one cognitive process, but should aim to combine several of them.
Behaviorism and eLearning
Behaviorism has inspired many of the types of eLearning styles and technologies with which we’re most familiar, from eLearning in its early incarnation as Computer-Assisted Instruction (CAI) to current-day page-turners and drill-and-practice games. Behaviorist technologies typically have explicit and discrete steps and are, therefore, amenable to automation. Figure 2.4 shows an example of an early “teaching machine” based on the behavioral approach.
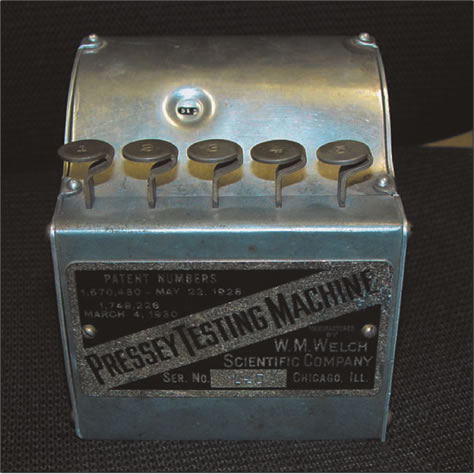
CAI, for example, provides guided individual learning and was initially designed for military training and language learning. CAI can also refer to software that involves a series of branching steps designed by an instructional designer to ensure that the learner moves on to the next step only when ready or gets diverted to further instruction, depending on the accuracy of their response. Following a behaviorist view, learners would be asked to provide definitions verbatim.
Bloom’s taxonomy and behaviorist training approaches are prevalent in the modern workplace. For the same reason that CAI suited military training early on, the promise of low-cost efficiency and objectives structured around predictable behaviors continues to appeal for many types of job-skills training in modern organizations.
Learning based on behaviorism tends to focus on rote learning through drill and practice exercises. The learner (“the empty vessel”) is required to memorize then reiterate information to show learning. In CAI, learning material is often presented as small isolated chunks of knowledge with little emphasis on connecting the pieces.
Cognitivism: Mind as Computer
Just because we can’t see something easily enough to measure it, doesn’t mean it isn’t important. Behaviorism could not explain all the situations in which our actions stem from the workings of our mind—not just our environment. This gap in behaviorism lead to the development of the theory of cognitivism in the 1950s. Dismissing the behaviorists’ view of the mind as an inaccessible black box, proponents of cognitive theory began to seek ways to understand the mind itself, and they found their answer in the emerging field of computer science.
Cognitivism is based on the idea that our minds can be understood as computers that process information. MIT Professor and artificial intelligence pioneer Marvin Minsky put it graphically when he said our mind is “a meat machine.” Cognitivists sought to model the human brain accurately on the assumption that this would help us design instruction for more complex behavior than behaviorism allowed—like problem solving and decision making.
Robert M. Gagné and Instructional Design
Theory longs to be applied and models make this possible. It’s the models, strategies and approaches used for teaching which allow theories to take shape in the form of real-world programs.
The most popular collection of models and strategies in eLearning can be herded under the umbrella of Instructional Design. Instructional Design (ID) is a field with a multitude of practices which were historically founded on behaviorist and cognitivist theories of learning. A central structure for ID is frequently summed up by the acronym ADDIE, which stands for analysis, design, development, implementation, and evaluation.
Instructional design can be traced back to World War II when psychologists like Robert Gangé were recruited to develop training programs for the military. The work that followed finally got a name in the 1960s when it was tagged with various labels, including instructional design, instructional systems design (ISD), and systematic instruction. By the end of the 1970s, an impressive 40 different models for systematically designing instruction had been defined. Through the ’80s, ID became particularly influential in business, industry, and military training.
A major influence in the field of Instructional Design was Robert Gagné who developed ideas that were hatched by behaviorism and took flight with cognitivism. He was an American Instructional Psychologist (1916–2002) and author of the book, The Conditions of Learning, with a first edition in 1965 founded on behaviorist strategies and later revisions that evolved into cognitive information processing. Both theories were well suited to a professional lifetime spent researching military training.
Like Bloom, Gangé is also famous for a taxonomy of learning outcomes. Gagné classified outcomes into five categories of learned capabilities: Intellectual Skills, Cognitive Strategy, Verbal Information, Attitude, and Motor Skills. Each of these is linked to a set of “conditions of learning” that form the basis of his theory of instruction.
He also provided strategies for facilitating these learning outcomes in the form of “nine events of instruction” intended to help transfer knowledge into learner memory.
Gagné’s 9 Events of Instruction
- Gain attention.
- Inform learners of objectives.
- Stimulate recall of prior learning.
- Present the content.
- Provide "learning guidance."
- Elicit performance (practice).
- Provide feedback.
- Assess performance.
- Enhance retention and transfer to the job.
Having such a tidy guide for effective learning has made Gagné’s work popular, particularly for professional development.
Cognitive Load—You Can Only Take in So Much at a Time
The cognitivist concept of cognitive load originated in discoveries on the limitations of our short-term memory—the human equivalent of a computer’s RAM. These findings produced the famous 7± 2 rule of working memory capacity—referred to as Miller’s law after the psychologist who suggested it.
The idea is that we can only keep about seven items in our working memory at any one time—like a phone number. When we’re faced with more than that, we manage it by chunking: aggregating the information into about seven chunks. More recently, researchers have actually put the limit at 4 ± 2.
Following on this growing understanding of working memory, Australian educational psychologist, John Sweller developed the concept of “Cognitive Load”. Cognitive Load describes the limitations of our working memory while we’re trying to learn something.
What has followed is a wealth of advice on how to free up learners minds for learning by reducing their cognitive load, or more specifically, their extraneous cognitive load—complexity that is not important for the learning task, but is added by the design or technology—for example, hard to read text; poorly written instructions.)
Cognitive Load Theory is also at the core of Richard Mayer’s work on multimedia learning, which comprises possibly the largest collection of research-based principles we have that deal specifically with multimedia design issues in education (see sidebar).
Schemas
Cognitivists assert that learning works better when we can connect new information to things we already know, and they call our existing mental framework for something a schema, or mental model. In Chapter 5, we’ll look at examples of visuals that support the integration of new knowledge via links to existing knowledge, including representational images, comparative images, and advance organizers.
Schemas provide a structure to which we can attach new information. Schemas are dynamic and change as we interpret new experiences and adapt our understanding accordingly.
Richard E. Mayer and Multimedia Learning
Many researchers turn to cognitive load theory to explain how we learn, but there is one stand-out for professionals in the area of multimedia learning and that’s American educational psychologist, Richard E. Mayer. His work is of special relevance to Learning Interface Designers because he has been dedicated to studying the impact of multimedia design on learning.
Based on cognitive load theory and findings from the cognitive sciences, Mayer and colleagues developed the Cognitive Theory of Multimedia Learning. This theory is based on three assumptions:
- We process visual and auditory information through separate channels—“dual-channel processing.”
- We are limited in the amount of information we can take into either channel at once.
- When we are engaged in active learning, we are not passively receiving information. Instead we a) pay attention, b) organize incoming information, picking and choosing what’s important, and c) integrate incoming information with other knowledge. We do all this in order to build a mental model of the key parts and relationships of the information we’re presented with.
These assumptions have implications for how multimedia learning environments and resources should be designed, and Mayer has spent over 15 years testing specific guidelines for multimedia learning design from the perspective of this theory. Specifically, he has studied the relative superiority of different combinations of text, graphics, video, and audio for different learning contexts. His research has lead to the development of a number of research-based multimedia learning design principles, many of which, but not all, pertain specifically to interface design.
Richard Mayer’s Principles of Multimedia Learning
- Multimedia principle
- Contiguity principle
- Modality principle
- Coherence principle
- Personalization principle
- Redundancy principle
- Segmenting principle
- Pre-training principle
- Signaling principle
- Voice principle
- Image principle
- Individual differences principle
More details on the application of these principles, as they pertain to interface design, are included across the strategies within this book. They are fully elaborated in eLearning and the Science of Instruction by Clark and Mayer.
Cognitivism and eLearning
Cognitivism’s love affair with computer science made it an ideal candidate as a theory for educational technology. In 1970, Jaime Carbonell suggested that, through dialogue with a student, computers could act as teachers and not just tools for learning. Rather than just the predetermined questions, answers, and predefined pathways that made up behaviorist CAI technologies, Carbonell envisioned “programs that know what they are talking about, the same way human teachers do.”
In motion toward this goal, CAI programs soon evolved into the more complex Intelligent Tutoring Systems (ITS). Ideally, ITS adapt to an individual student’s performance automatically by drawing on knowledge incorporated into their database. They are also intended to transfer lesson content to the learner, just as topic knowledge might be passed from tutor to student.
ITS have become more and more sophisticated, and some experimental examples even have capacity to detect and respond to learners’ emotional states. But, for a variety of reasons, they have not been widely adopted. We look at ITS more closely in the next chapter.
The Cognitive Sciences
In the last two decades of the 20th century, cognitive psychology expanded into the more multidisciplinary field of the cognitive sciences. Studies carried out by those working in the cognitive sciences brought many new understandings of learning to the table, including new ideas about knowledge, learning, and problem solving.
For example, key to cognitive-science study is the idea that we develop representations, or knowledge structures, in our mind—for example, concepts, beliefs, facts, procedures, models. Cognitive science also helped uncover the importance of reflection to learning and to expert behavior. Studies found that experts—as opposed to novices in a field—were better at the reflective practices of criticizing and planning their work. Thus, novices should have these abilities developed if they are to evolve into experts.
Cognitive science has given learning theory an injection of sociocultural perspective as well. Socioculturalists study learning outside of schools and outside of Western culture. Their work has revealed that, outside of schools, learning almost always takes place within a complex social environment and, therefore, learning can’t be fully understood as a mental process taking place only within the boundaries of someone’s head. Their physical and social environment must also be considered. These results fueled the theory of situated cognition (described below) and align with the descriptions of learning provided by constructivism.